In customer education circles, conversations often loop back to the same existential question: If we believe that CE is so valuable, why is it so hard to prove it?
Daniel Quick, Head of Customer Education at Asana, wanted to leverage Asana’s experimentation culture to demonstrate how his team’s work improved outcomes.
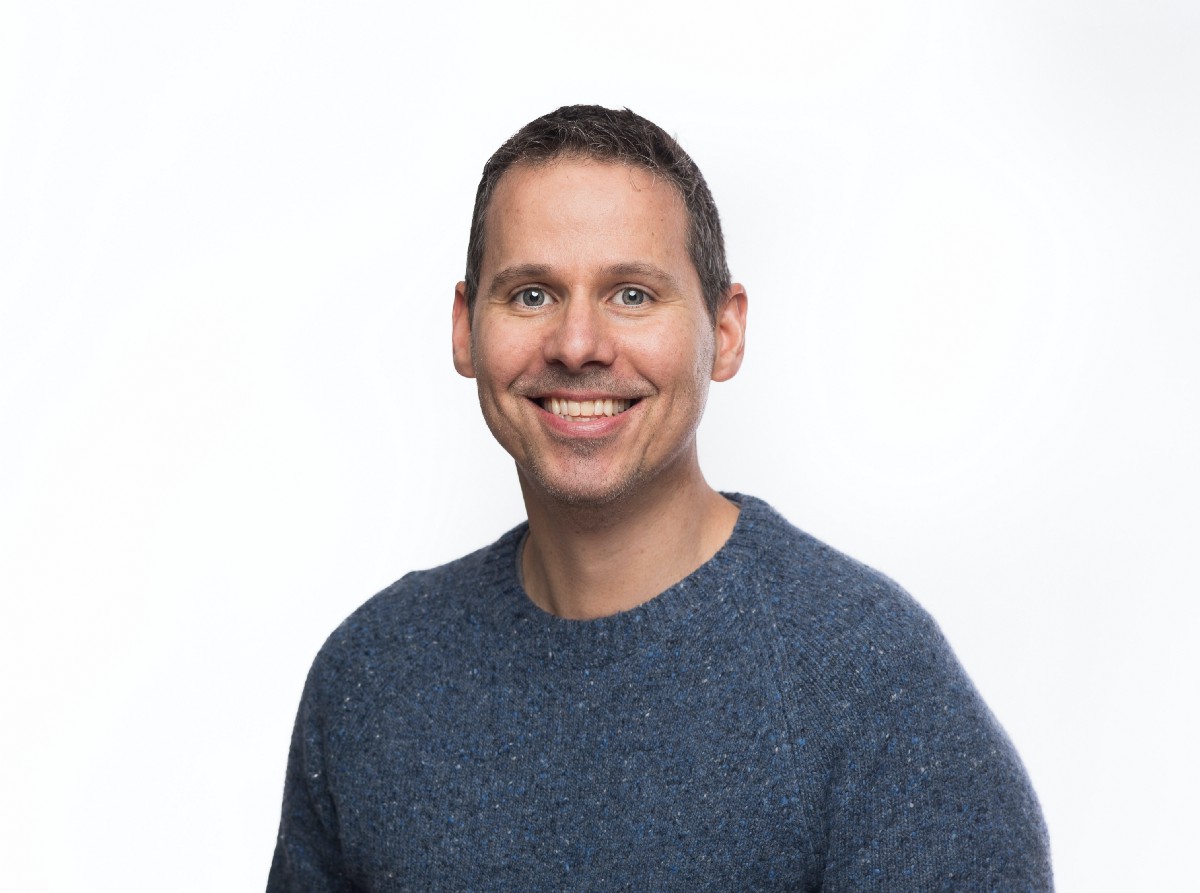
“Asana is a data-driven business,” said Daniel. “It’s important for us to understand the impact of the work we do on our customers’ experience.”
Recently, Asana ran an experiment with customer success, A/B testing a “top bar” that promoted a customer success coaching session to unlock the power of Asana. The experiment demonstrated that users who saw the bar engaged more often with the CS team, which — in turn — led to downstream adoption and utilization.
Daniel wondered if he could create a similar experiment for the education team. To his thinking, crafting learning experiences for customers is hard; shouldn’t running the experiments be easy?
Correlation vs. Causation in Customer Education
Let’s start with one hard truth:
📌 Running statistically significant experiments to prove the value of customer education is challenging and rarely done.
Although it is relatively easy to correlate engagement with training and content with user success, causation is much harder to prove. Who’s to say that it was the training and not some other factor that caused higher performance among users who engaged vs. those who didn’t
So, what makes running causation experiments in CE so tough?
- Small N: Often, we have relatively small numbers of users to test. This is particularly true for those using email campaigns, as your N decreases rapidly once you factor in open and click-through rates.
- The Motivated Learner: In a sad irony, the users that are most likely to seek out training are those that are least likely to need it. This can bias our sample set toward a group of motivated learners.
- Scarce Resources: Sales, marketing, customer success, customer education, and product teams are all trying to run tests, but users only notice so many pop-ups or emails before it becomes noise, or, worse yet, degrades the experience.
- Multiple Systems: Critical data is often stored across systems. For example, one system may hold educational content, another training data, and a third in-app production data. Attempting to create a coherent dataset across these sources can be a herculean task!
- Multiple Users (per Account): While individual users consume training, it’s often the account that we care about. Is an account sufficiently trained if only one user consumes training? What happens when that user switches teams or leaves the company? Often, there is a mismatch between which users have been trained and metrics we aim to move (e.g., account MRR).
- The Sequencing Problem: Which came first — the training, the feature adoption, or the support contact? We may be able to demonstrate that users who engage with training are more likely to be power users, but it can be tricky to show that their usage increased only after they engaged with training. Although we may have instrumented the feature we want to track, we may neglect to capture the historical event state (i.e., how confident are we that our user never completed a similar event before the training took place?).
- Inferring Comprehension: Often, we hold up feature usage as our North Star metric. Yet, clicking does not always indicate comprehension. A trigger-happy user may access a new feature without understanding its value. Many learning management solutions contain “concept checks” (e.g., mini-quizzes), though these are rarely used in product!
- Lack of Control Group: Last, but not least, to understand causation, our experiment needs a control group of users who did not receive any treatment. However, it’s uncommon for a company to not offer its training or its content in order to prove the impact. As the saying goes, if you got it, flaunt it.
😔 Phew! So, what is a data-driven customer educator to do?
Running Customer Education Experiments the Right Way
Much like with Asana’s customer success experiment, Daniels wants to take advantage of in-app messaging to run a causal experiment. Here’s how:
- Bigger N: Because Asana shows its messaging in-app, it has access to its full set of users, not just those who open their Asana emails.
- The Motivated & Unmotivated Learner: The experiment is a blind A/B test, so motivated and unmotivated users alike will have equal access to the content.
- Plentiful Resources: Asana’s Product team created a queue for running experiments and a braintrust of leaders to manage those interactions so that each experiment could run independently.
- Multiple Connected Systems: Asana has a history of running experiments and has built a data pipeline that connects each of its systems to track the results.
- Sufficient Scope: This experiment is scheduled to run for several weeks, enough time to absorb multiple product changes so that changes in user behavior can be attributed back to the customer education campaign.
- Measuring Utilization + Adoption: Asana uses multiple metrics — a composite utilization metric to assess account health, measuring the percent of users on an account that regularly login, and an adoption metric to track the individual user behavior. Combined, these two metrics give Asana a good sense of whether any change moved the needle.
- A Control Group: Asana was willing to run a blind A/B test across its entire user base. Half of its users received the custom messaging, and the other half received nothing at all, giving Asana a large enough control group to get statistically significant results.
No one ever said it would be easy, but Daniel could finally unpack how awareness of the educational resources in Asana Academy generate downstream adoption and retention. Stay tuned for the results!